Greetings! I'm Aneesh Sreedharan, CEO of 2Hats Logic Solutions. At 2Hats Logic Solutions, we are dedicated to providing technical expertise and resolving your concerns in the world of technology. Our blog page serves as a resource where we share insights and experiences, offering valuable perspectives on your queries.
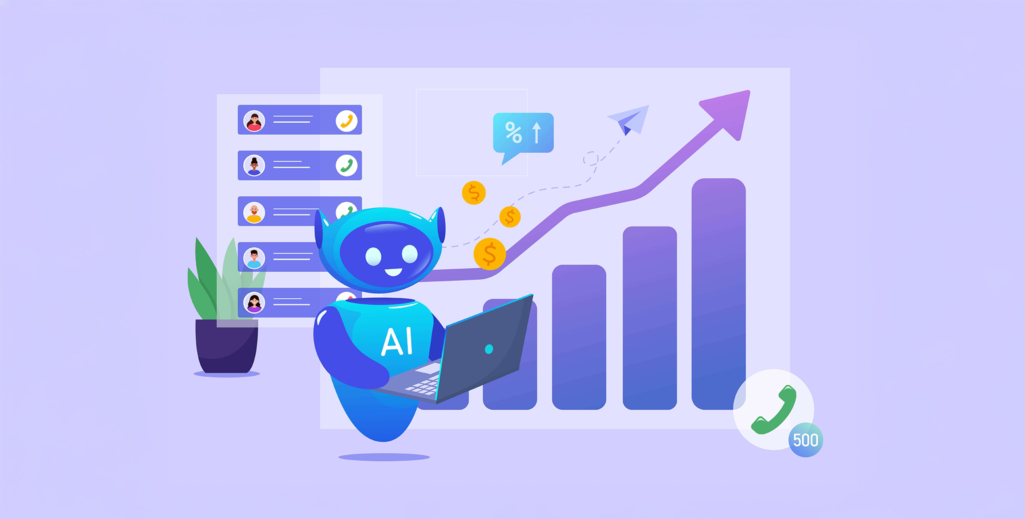
Quick Summary
This article explores how artificial intelligence is revolutionizing e-commerce sales forecasting, helping businesses optimize inventory, pricing strategies, and marketing efforts through advanced data analysis. We’ll examine practical applications, implementation strategies, and the tangible benefits of AI-powered sales prediction for online retailers of all sizes.
E-commerce success now hinges on a single critical capability: accurately predicting what will sell tomorrow.
Retailers who master sales forecasting outperform competitors by 15-30% in profitability, transforming prediction accuracy directly into market dominance.
Leading retailers now deploy AI to answer their most valuable questions: Which products will trend next month?
How will pricing changes impact category demand? When should seasonal inventory arrive? What regional factors will drive sales variations?
Forward-thinking companies aren’t just adopting AI, they’re rebuilding their entire operational models around its predictive capabilities, creating responsive systems that anticipate consumer demand before it fully materializes.
Pro Tip: Even small e-commerce businesses can benefit from AI-powered sales prediction. You don’t need enterprise-level resources to implement basic machine learning models that can significantly improve your forecasting accuracy.
Why Traditional Sales Forecasting Falls Short
Traditional sales forecasting methods typically rely on historical data analysis, seasonal trends, and human intuition. While these approaches have served businesses for decades, they come with significant limitations in the digital age:
- They struggle to process the vast volumes of data generated by e-commerce platforms
- They can’t effectively account for rapidly changing market conditions
- They miss complex patterns and correlations that influence purchasing behavior
- They’re slow to adapt to emerging trends and consumer preferences
- They lack the precision needed for optimized inventory management
These limitations can lead to costly errors: overstocking products that gather dust in warehouses or understocking popular items and missing sales opportunities.
In the world of e-commerce, these inefficiencies can quickly erode profit margins and damage customer satisfaction.
Warning: Relying solely on basic historical data analysis for your e-commerce forecasting puts you at risk of missing critical sales opportunities and making costly inventory mistakes in today’s dynamic market environment.
How AI Transforms E-commerce Sales Prediction
Artificial intelligence brings a new level of sophistication to sales forecasting through its ability to:
1. Process Massive Datasets
AI systems can analyze millions of data points across multiple channels and sources, identifying patterns invisible to human analysts. These include:
- Historical sales data across all products and categories
- Website traffic and user behavior analytics
- Seasonal trends and cyclical patterns
- Social media engagement and sentiment
- Economic indicators and market conditions
- Competitor pricing and promotional activities
2. Identify Complex Patterns
Using techniques like machine learning and deep learning, AI can detect subtle correlations between seemingly unrelated factors. For example, an AI system might discover that sales of certain products spike three days after specific weather conditions in particular regions, a pattern too complex for traditional analysis to uncover.
3. Deliver Real-time Insights
Unlike traditional forecasting that often operates on monthly or quarterly cycles, AI systems can continuously analyze incoming data and update predictions in real-time, allowing businesses to respond immediately to changing conditions.
4. Improve Over Time
Perhaps most importantly, AI systems learn from their successes and failures. As they process more data and observe actual outcomes, their predictive accuracy continuously improves through a process called machine learning.
Pro Tip: When implementing AI for sales prediction, start with a specific business challenge (like reducing overstock of seasonal items) rather than attempting to overhaul your entire forecasting system at once. This targeted approach allows you to demonstrate clear ROI before expanding.
Key AI Technologies Driving E-commerce Sales Prediction
Several AI technologies are particularly relevant for e-commerce sales forecasting:
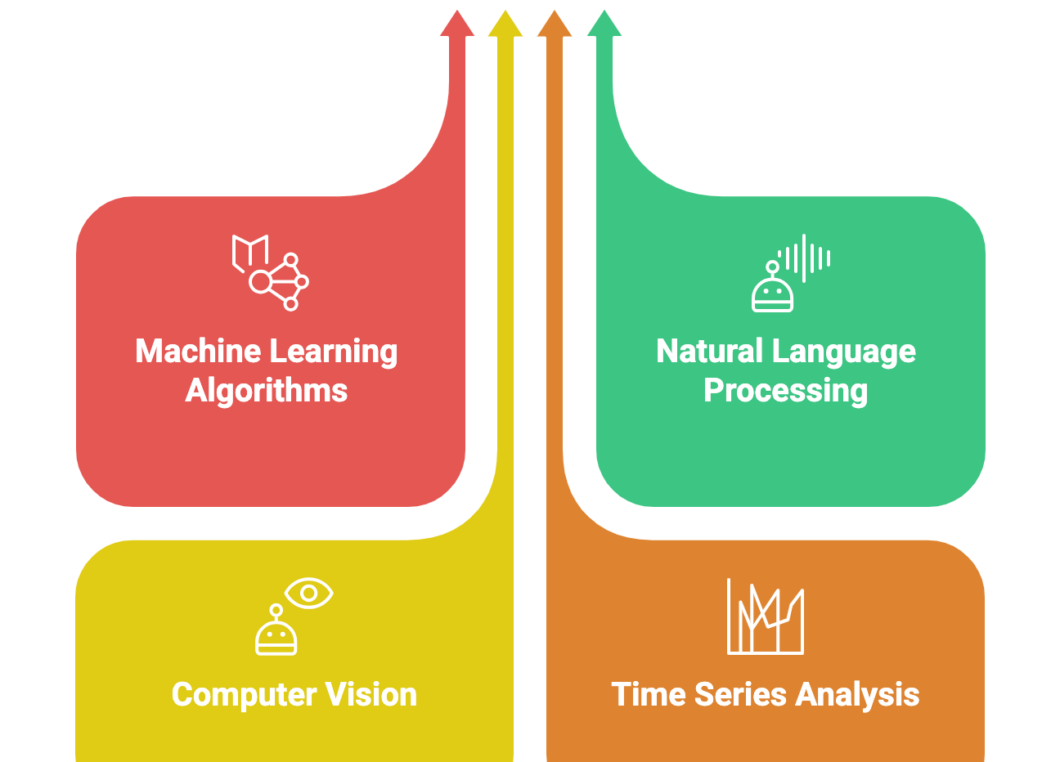
Machine Learning Algorithms
Machine learning models like random forests, gradient boosting, and neural networks can identify patterns in historical sales data and use these insights to make future predictions.
These algorithms excel at handling the complex, multidimensional data typical in e-commerce environments.
Natural Language Processing (NLP)
NLP allows AI systems to analyze customer reviews and social media posts, as well as support interactions to gauge sentiment and identify emerging trends or issues that might impact sales.
This technology provides valuable context beyond pure transactional data.
Computer Vision
For fashion and home décor retailers, computer vision can analyze visual trends across social media and other channels to predict which styles and designs are gaining popularity, informing inventory and merchandising decisions.
Time Series Analysis
Specialized AI models designed specifically for time-based data can detect seasonal patterns, trends, and anomalies in sales histories, providing highly accurate short and medium-term forecasts.
Not sure which AI technologies would best suit your e-commerce business?
Implementing AI for E-commerce Sales Prediction
Transforming raw data into actionable sales forecasts requires a systematic approach that balances technical capabilities with clear business objectives.
Here’s how to build your AI prediction engine from the ground up.
Step 1: Audit Your Data Resources
Before implementing any AI solution, assess the quality and accessibility of your data:
- Sales history (ideally at least 2 years of data)
- Product information and attributes
- Customer demographics and behavior data
- Marketing campaign performance
- External factors (economic indicators, seasonality, competitor activities)
The success of any AI prediction model depends heavily on data quality. Ensure your data is accurate, consistent, and properly structured before proceeding.
Step 2: Define Clear Objectives
Determine exactly what you want to predict and why. Common objectives include:
- Daily/weekly/monthly sales forecasts by product category
- Inventory requirements for upcoming seasons
- Impact of pricing changes on sales volume
- Customer lifetime value predictions
- Churn risk and retention opportunities
Having specific goals will guide your choice of AI models and implementation approach.
Step 3: Choose Your Implementation Path
Depending on your resources and technical capabilities, you have several options:
- Built-in tools: Many e-commerce platforms now offer integrated AI prediction features
- Third-party solutions: Specialized software providers offer plug-and-play AI forecasting tools
- Custom development: For larger businesses, developing proprietary AI models may be worthwhile
- Hybrid approach: Combining off-the-shelf solutions with customized elements
Warning: Don’t underestimate the importance of data preparation when implementing AI sales prediction. Even the most sophisticated algorithms will deliver poor results if fed with inconsistent or incomplete data.
Step 4: Start Small and Scale
Begin with a pilot project focused on a specific product category or business challenge. This approach:
- Minimizes initial investment and risk
- Allows you to demonstrate ROI before scaling
- Provides valuable learning opportunities
- Helps build organizational buy-in
Once you’ve proven the concept, gradually expand to other areas of your business.
Step 5: Integrate with Existing Systems
For maximum impact, ensure your AI predictions feed directly into your:
- Inventory management system
- Marketing automation platform
- Pricing optimization tools
- Customer service systems
This integration enables automatic actions based on AI insights, rather than requiring manual intervention.
Real-World Applications of AI Sales Prediction in E-commerce
By predicting which products individual customers are most likely to purchase next, AI can power highly effective recommendation engines that boost average order value and conversion rates.
Example: Fashion retailer StyleNow uses AI to analyze purchase history, browsing behavior, and style preferences to create personalized home pages for each visitor, resulting in a 34% increase in conversion rate.
Pro Tip: When using AI for inventory optimization, don’t just focus on avoiding stockouts. The cost savings from preventing overstock situations often deliver even greater ROI, especially for products with higher storage costs or seasonal demand patterns.
Measuring the Impact of AI on E-commerce Sales Prediction
To evaluate the effectiveness of your AI sales prediction implementation, track these key metrics:
Forecast Accuracy
- Mean Absolute Percentage Error (MAPE)
- Root Mean Square Error (RMSE)
- Forecast Bias (tendency to over or under-predict)
Business Impact
- Inventory turnover rate
- Stockout frequency
- Carrying costs
- Markdown/discount frequency
- Marketing conversion rates
- Customer satisfaction scores
Regular benchmarking against previous forecasting methods will help quantify the ROI of your AI investment.
Ready to see how AI could improve your sales forecasting accuracy?
Overcoming Common Challenges in AI-Powered Sales Prediction
Even the most sophisticated AI implementation journeys encounter obstacles. Here are the primary hurdles businesses face when adopting predictive analytics and proven strategies to conquer them.
Data Silos and Integration Issues
Many e-commerce businesses struggle with fragmented data across multiple platforms and departments. Breaking down these silos is essential for comprehensive AI analysis.
Solution: Implement a data lake or warehouse strategy that centralizes information from all relevant sources before feeding it into your AI systems.
Resistance to Change
Stakeholders accustomed to traditional forecasting methods may resist adopting AI-powered predictions, especially if they don’t understand the technology.
Solution: Focus on transparency and explainability in your AI models, and demonstrate concrete benefits through small-scale pilot projects.
Handling External Disruptions
Unprecedented events (like pandemic-related lockdowns) can temporarily reduce the accuracy of AI predictions based on historical patterns.
Solution: Incorporate scenario planning capabilities and ensure your AI system can quickly adapt to new information and changing conditions.
Warning: Be wary of “black box” AI solutions that don’t provide visibility into how predictions are generated. Without understanding the factors driving forecasts, it’s difficult to build trust in the system or troubleshoot when predictions seem counterintuitive.
The Future of AI in E-commerce Sales Prediction
As technology continues to evolve, several emerging trends will shape the future of AI-powered sales forecasting:
Explainable AI
Next-generation models will provide clear explanations for their predictions, helping business users understand not just what will happen, but why.
Cross-Channel Integration
AI systems increasingly incorporate data from physical stores, social commerce, voice shopping, and other emerging channels to create unified predictions across the retail ecosystem.
Autonomous Decision-Making
Beyond simply providing predictions, advanced AI systems will automatically execute inventory, pricing, and marketing decisions based on forecasted outcomes.
Predictive Customer Service
AI will anticipate customer service needs before they arise, allowing e-commerce businesses to proactively address potential issues that might impact sales.
Pro Tip: When calculating ROI for your AI implementation, don’t overlook “soft” benefits like reduced forecasting time for your team, improved supplier relationships due to more accurate ordering, and decreased stress during seasonal peaks due to better preparation.
Conclusion
AI-powered forecasting isn’t just about improving operational efficiency, it’s about creating a responsive, data-driven business capable of anticipating customer needs and market shifts before they happen.
Whether you’re just beginning to explore AI capabilities or looking to enhance existing predictive systems, the journey toward data-driven sales forecasting is both necessary and rewarding.
By starting small, focusing on concrete business objectives, and gradually expanding your AI capabilities, you can transform your approach to sales prediction and unlock new levels of growth and profitability in your e-commerce operations.
See how data-driven insights can optimize your inventory, pricing, and marketing decisions
FAQ
How accurate are AI sales predictions compared to traditional methods?
Most e-commerce businesses implementing AI forecasting report accuracy improvements of 20-50% compared to traditional methods. However, results vary depending on data quality, industry, and specific use cases. The greatest accuracy gains typically occur for businesses with highly seasonal products or complex inventory challenges.
What size business benefits most from AI sales prediction?
While enterprise retailers have been early adopters, mid-sized e-commerce businesses (with annual sales of $5-50 million) often see the most dramatic ROI from AI implementation. These companies have sufficient data volume to train effective models while facing inventory and forecasting challenges that AI can readily solve.
How much historical data is needed for effective AI predictions?
Generally, AI systems perform best with at least 2 years of historical data to account for seasonal patterns. However, even with 6-12 months of data, many AI models can deliver useful predictions, especially for fast-moving product categories where recent trends matter more than long-term patterns.
Can AI predict sales for new products with no sales history?
Yes, through a technique called "similarity modeling" or "cold-start prediction." AI can analyze how similar products performed during their launches and extrapolate likely outcomes for new products based on shared attributes, target audience, and market conditions.
Table of contents
- Why Traditional Sales Forecasting Falls Short
- How AI Transforms E-commerce Sales Prediction
- Key AI Technologies Driving E-commerce Sales Prediction
- Implementing AI for E-commerce Sales Prediction
- Real-World Applications of AI Sales Prediction in E-commerce
- Measuring the Impact of AI on E-commerce Sales Prediction
- Overcoming Common Challenges in AI-Powered Sales Prediction
- The Future of AI in E-commerce Sales Prediction
- Conclusion
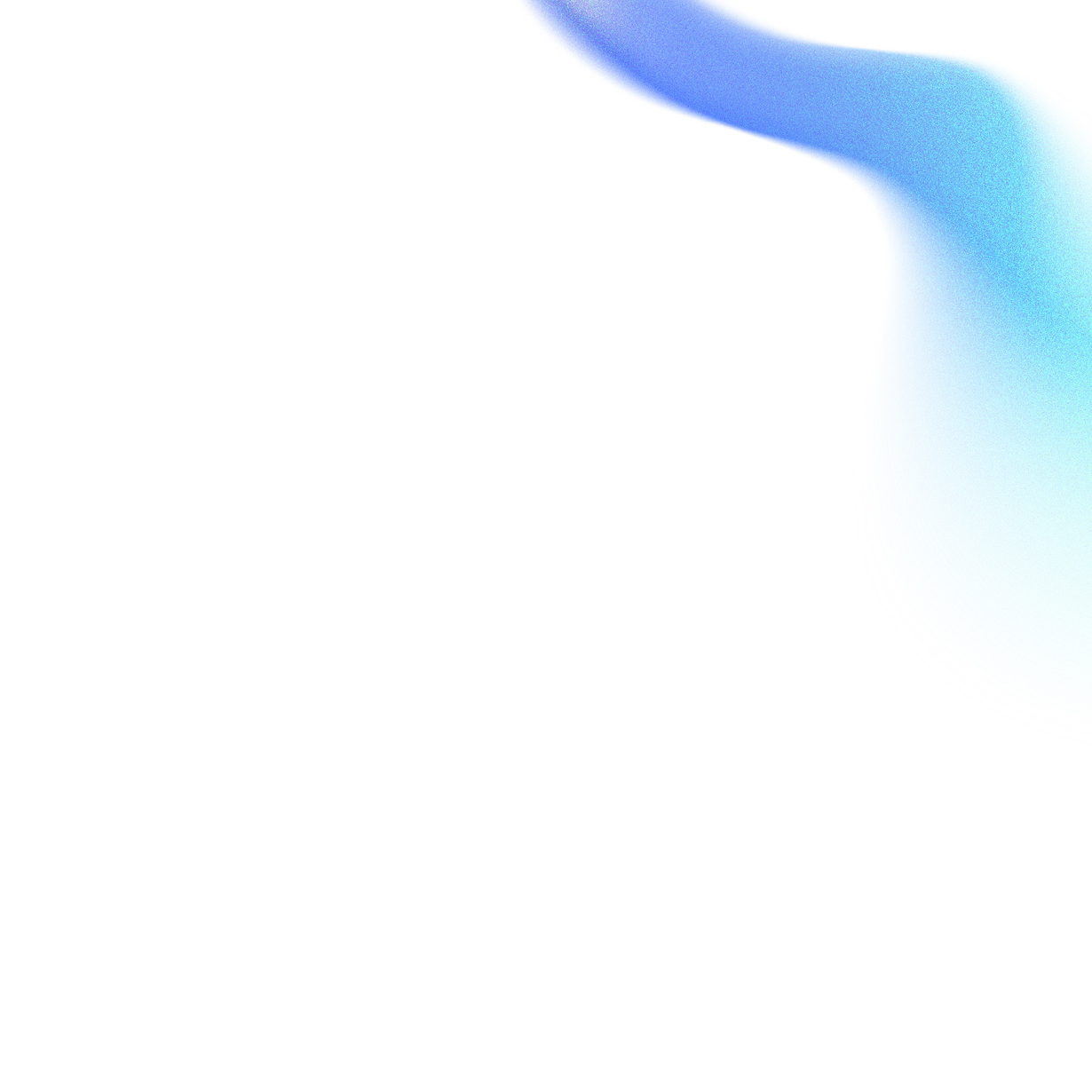
Related Articles
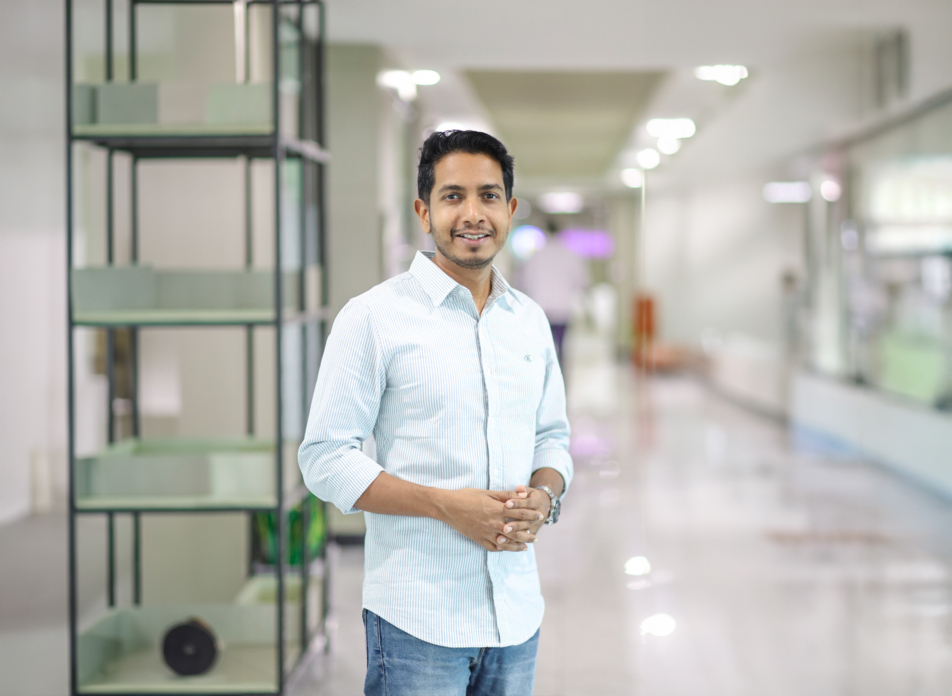